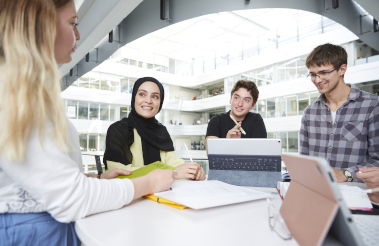
Second round: We are again calling researcher tandems (MATH and SEE) at KIT to submit interdisciplinary project ideas!
Deadline: September 12, 2025
Learn more (only visible in KIT intranet)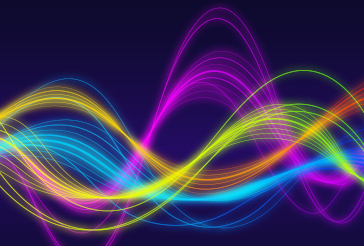
Neural Operators, Gaussian Processes and Application Day
Date
August 27-29, 2025
Venue
Campus South, Building 20.30
Room 0.014
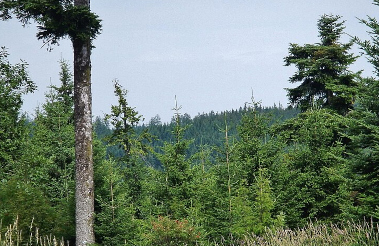
Scientific exchange and networking in the Black Forest
Date
November 10-12, 2025
Venue
Naturfreundehaus Kniebis, Black Forest
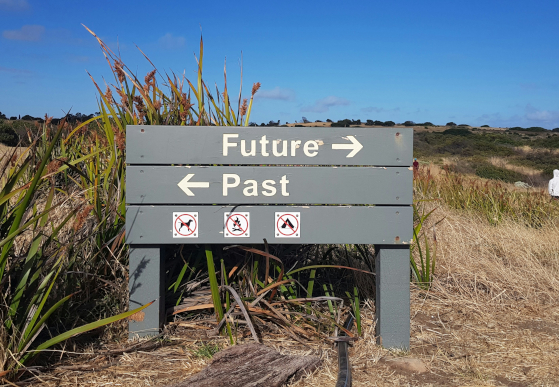
Find an overview of past events in our timeline.
linkEvent Calendar
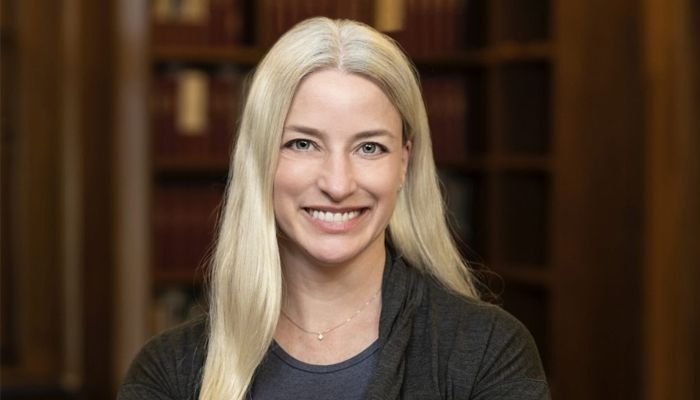
KIT International Excellence Fellow Prof. Victoria Stodden invited the KIT community, especially early career researchers, to create ideas and proposals for facilitating research that is data-, compute-, or AI-enabled - taking reproducibility to action! The workshop took place on January 21, 2025 at Triangel Studio.
Read moreFrom Computational and Data Science to Industry and Academia - four KIT Alumni gave insights into their jobs during a lively panel discussion on October 11, 2024 at Triangel Space.
Read more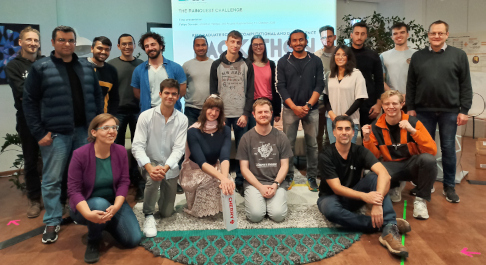
The hackathon on precipitation estimation with weather radar and rain gauge data took place from October 8-11, 2024 at Triangel Studio.
Read moreThe workshop with Dr. Annika Oertel (IMKTRO) and Dr. Vandana Jha (SCC) took place from September 11-12, 2024 at the Mathematics building.
Read more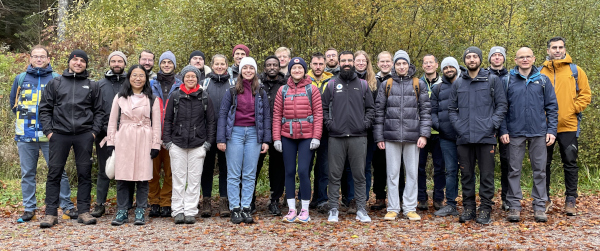
The second annual KCDS Retreat took place from November 13-15, 2023 at Naturfreundehaus Kniebis in the Black Forest.
Read more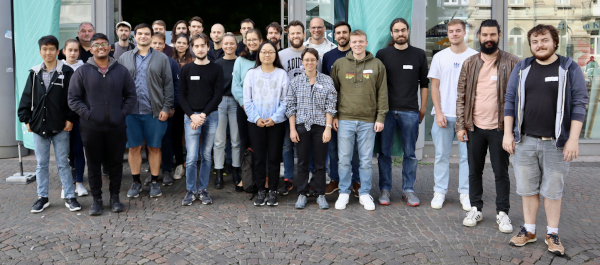
The workshop with a focus on "Recent Advances in Kernel Methods for Neural Networks" took place in October 5-6, 2023 at the Triangel.
Read more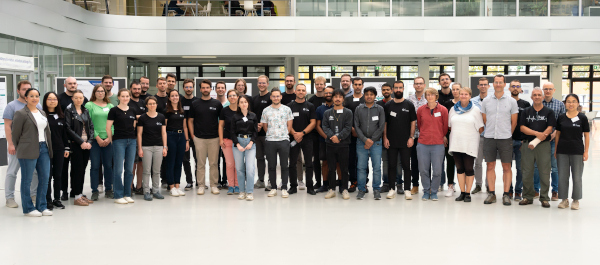
The first KCDS Summer School centered on the topic of Stochastic and Hybrid Modelling and took place at KIT Campus South, September 18-20, 2023.
Read moreAbout KCDS
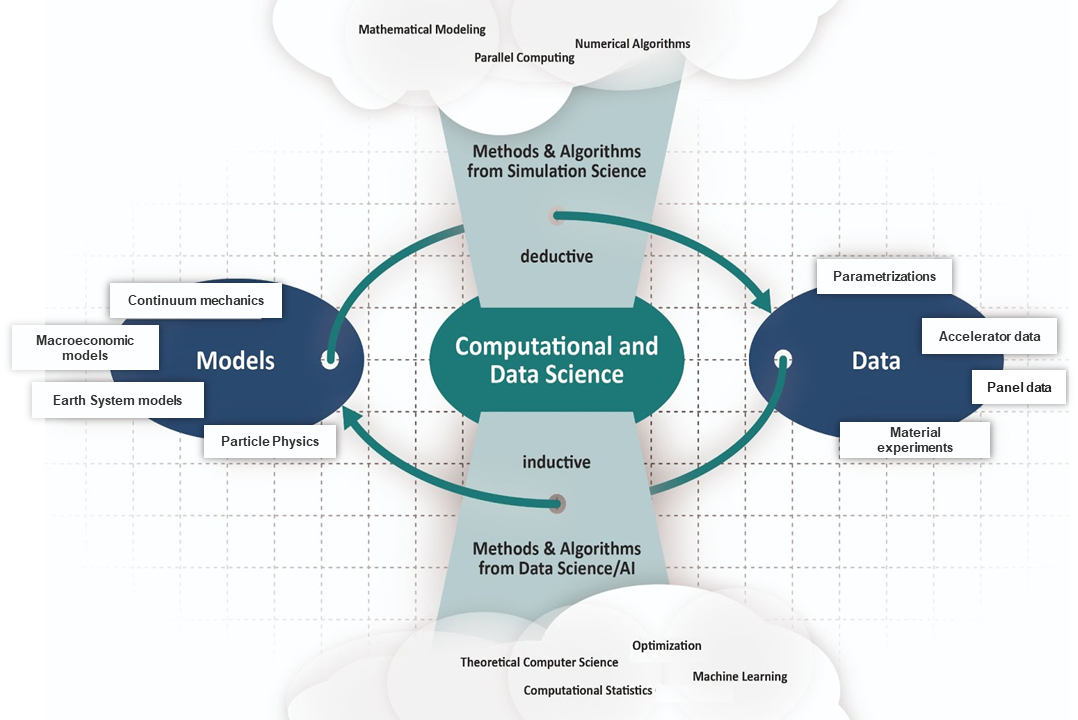
Coordination KIT Graduate School Computational and Data Science
+49 721 608-47748
angela huehnerfuss ∂does-not-exist.kit edu
www.kcds.kit.edu/